The Power of Direct Real-Time Feedback for Atomic-Scale Engineering
March 20, 2025
Blog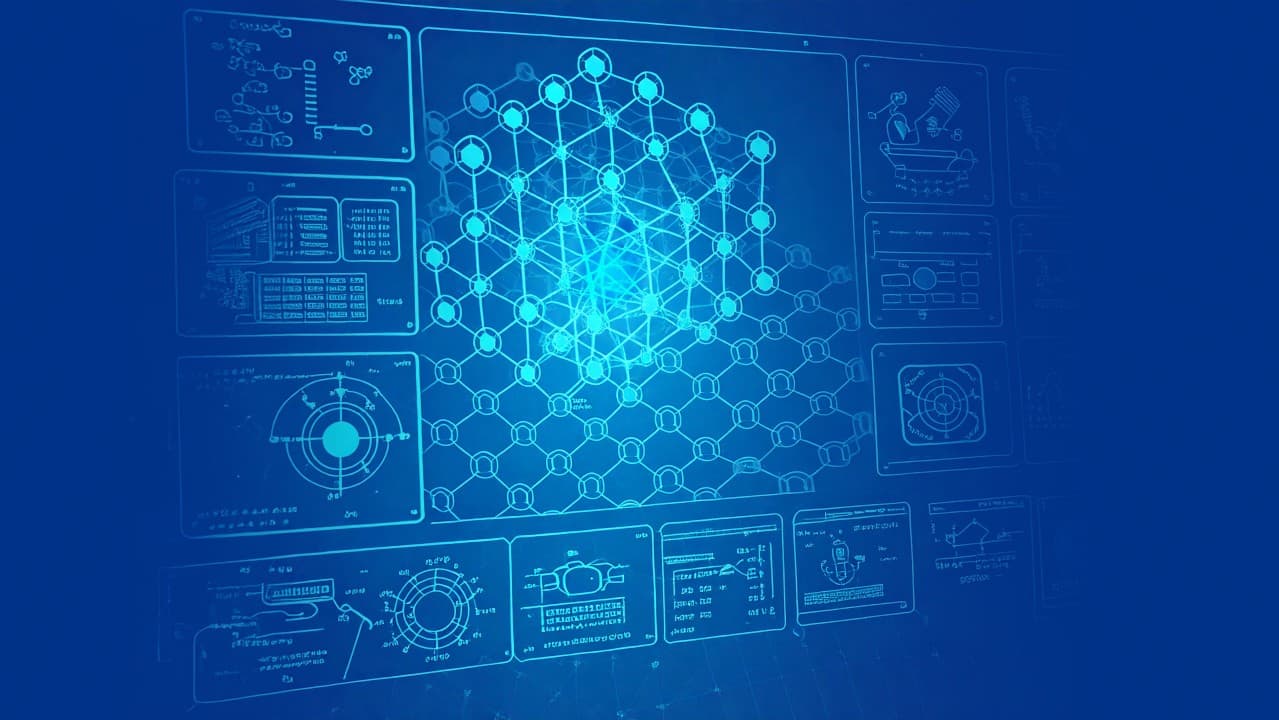
Atomic-scale engineering aims to create high-performance and high-value materials by controlling the assembly of matter with extreme precision. From discovering and synthesizing a new material for the first time to optimizing high-volume materials processing yields, progress requires measuring ground-truths directly from the materials of focus. Despite the critical importance of these ground-truths, they are currently generated through complex manual analysis of specialized data – outputs from tools that directly probe atomic-scale properties (materials characterization).
The way we currently generate ground-truth feedback from characterization results in two major consequences that hold back materials development and scale-up:
1. Blind spots: Direct materials characterization is difficult to use dynamically in-process, creating substantial blind spots for scientific and engineering aims and a reliance on batch-level iteration.
- Without direct-feedback in-situ, the evolution of a material in-process is left largely unobserved and unmeasured.
- When in-situ measurements are made, they are interpreted visually or with single-point analysis used to make qualitative inference. Signal-to-noise ratio is low and only a small fraction of the collected data is used.
- Tool telemetry is used to control and monitor in-process conditions, but these are proxies that are several degrees of separation from the material itself. The mapping between these variables and the materials state is not known and not simulable.
- Theory and past experience are extended as working assumptions to span the gap between the recipe (machine instructions) and the resulting material – inducing caution and a need to stay close to what is known.
- The lack of direct real-time feedback and consistent records of material processing deprives scientists and engineers of the opportunity to improve each step directly and explore a vast potential of dynamic, reactive process interventions.
2. Rate Limits: Batch-level iteration introduces practical limits for the rate of development in new materials and scale-up to economic production.
- Both the time required to complete batch processing and the time required to process ex-situ characterization have so far set practical limits on the cycle-time of scientific and materials engineering progress.
- Statistical methods for optimizing trial selection can reduce the number of batches required to explore a design / optimization space, but can't overcome roadblocks from the underlying approach.
- The minimum unit of experimentation (one batch) constrains the process design space. Scientists and engineers can't feasibly explore higher-resolution processing sequences because the combinatorics explode when each trial is evaluated as a whole, constraining the process design space.
- Shrinking the unit of experimentation and unit of feedback from the batch and allowing it to scale to the individual process steps is the only way to break down these structural limitations – real-time, direct material feedback is one of the most promising ways to achieve this.
An AI system for direct real-time feedback based on current tools.
Our breakthrough technology, demonstrated for in-situ diffraction and generalizable to other characterization approaches, uses custom AI to enable direct real-time materials feedback with existing hardware, upending these challenges and introducing a new era for atomic scale engineering. We remove blind spots by combining flexible real-time data recording UX with the ability to parse complex materials characterization data into interpretable outputs at scale. We generate the most quantitatively comprehensive fingerprints for atomic-scale resolution and deliver them in time to make process adjustments, providing a new foundation for process control based on direct, ground-truth material feedback.
Automatically generated, high-resolution direct materials feedback reduces the minimum unit of experimentation to explore cause and effect in real-time, providing an effective platform to map correlated external measurements to in-process monitoring. This removes the rate-limit of batch iteration and allows us to introduce dynamic monitoring, alerting, and intervention generation with teams of context-aware AI agents. Agents leverage the general reasoning ability of large language models with the unique and specific context provided by our materials feedback engine. They produce outputs in natural language for human-in-the-loop systems or as structured text to interface directly with equipment APIs. Most importantly, they enable processes designed on a foundation of direct materials feedback to be automated and scaled as they monitor live streams of incoming results.
The convergence of in-situ hardware, applied AI for materials science, and general foundation models gives us the tools to build a new low-level programming language for the physical world. Atomic Data Sciences is delivering the first end-to-end AI solution for advanced materials synthesis.